チュートリアル3:Cortex Searchで PDF チャットボットを構築する¶
概要¶
このチュートリアルでは、Cortex Searchを使って、 PDF ドキュメントのデータセットからチャットボットを構築する方法を説明します。 チュートリアル 2 では、すでにソースから抽出されたテキストデータからチャットボットを構築する方法を学びました。このチュートリアルでは、基本的なPython UDF を使って、 PDFs からテキストを抽出し、抽出されたデータをCortex Search Serviceに取り込む例を説明します。
学習内容¶
Python UDF を使って、ステージ内の PDF ファイルのセットからテキストを抽出します。
抽出したテキストからCortex Search Serviceを作成します。
Streamlit-in-Snowflake チャットアプリを作成し、 PDF ドキュメントから抽出したデータについて質問できるようにします。
前提条件¶
このチュートリアルを完了するには、以下の前提条件が必要です。
データベーステーブル、仮想ウェアハウスオブジェクト、Cortex Search Service、およびStreamlitアプリを作成するために必要な権限を付与するロールを持つSnowflakeアカウントとユーザーを持っています。
これらの要件を満たす手順については、 Snowflakeを20分で紹介 をご参照ください。
ステップ1: 設定する¶
PDF データの取得¶
このチュートリアルでは、連邦公開市場委員会(FOMC)の議事録のサンプルデータセットを使用します。これは、2023年と2024年に開催された FOMC 会議のメモを含む10ページにわたる12のドキュメントのサンプルです。このリンクをクリックして、ブラウザから直接ファイルをダウンロードします。
FOMC の議事録一式は、 US 連邦準備制度理事会(FRB)のウェブサイト で閲覧できます。
注釈
チュートリアル以外の設定では、自分のデータ(おそらくすでにSnowflakeステージにあります)を持ち込むことになります。
データベーステーブル、ウェアハウスの作成¶
次のステートメントを実行して、データベース、2つのテーブル(csvおよびJSONデータ用)、およびこのチュートリアルに必要な仮想ウェアハウスを作成します。チュートリアルの完了後に、これらのオブジェクトをドロップできます。
CREATE DATABASE IF NOT EXISTS cortex_search_tutorial_db;
CREATE OR REPLACE WAREHOUSE cortex_search_tutorial_wh WITH
WAREHOUSE_SIZE='X-SMALL'
AUTO_SUSPEND = 120
AUTO_RESUME = TRUE
INITIALLY_SUSPENDED=TRUE;
USE WAREHOUSE cortex_search_tutorial_wh;
注釈
CREATE DATABASE
ステートメントはデータベースを作成します。データベースには、 PUBLIC という名前のスキーマが自動的に含まれます。CREATE WAREHOUSE
ステートメントは、中断された初期状態のウェアハウスを作成します。
ステップ2: データをSnowflakeにロードする¶
まず、データを含むファイルを格納するSnowflakeステージを作成します。このステージでは、会議の議事録 PDF ファイルが保管されます。
CREATE OR REPLACE STAGE cortex_search_tutorial_db.public.fomc
DIRECTORY = (ENABLE = TRUE)
ENCRYPTION = (TYPE = 'SNOWFLAKE_SSE');
注釈
ディレクトリと暗号化は、ファイルのpresigned_urlを生成するために設定されます。presigned_urlを生成する必要がない場合は、これらの設定を省略することができます。
データセットをアップロードします。データセットのアップロードは、 Snowsight または SQL を使用します。 Snowsight にアップロードするには:
Snowsight にサインインします。
左側のナビゲーションメニューで Data を選択します。
データベース
cortex_search_tutorial_db
を選択します。スキーマ
public
を選択します。Stages 、
fomc
の順に選択します。右上の + Files ボタンを選択します。
ファイルを UI にドラッグ&ドロップするか、 Browse を選択してダイアログウィンドウからファイルを選択します。
Upload を選択してファイルをアップロードします。
ステップ 3: PDF ファイルを解析する¶
次のような前処理関数を作成します。
PDF ファイルを解析し、テキストを抽出します。
索引付けのためにテキストをチャンクに分割にします。
CREATE OR REPLACE FUNCTION cortex_search_tutorial_db.public.pdf_text_chunker(file_url STRING)
RETURNS TABLE (chunk VARCHAR)
LANGUAGE PYTHON
RUNTIME_VERSION = '3.9'
HANDLER = 'pdf_text_chunker'
PACKAGES = ('snowflake-snowpark-python', 'PyPDF2', 'langchain')
AS
$$
from snowflake.snowpark.types import StringType, StructField, StructType
from langchain.text_splitter import RecursiveCharacterTextSplitter
from snowflake.snowpark.files import SnowflakeFile
import PyPDF2, io
import logging
import pandas as pd
class pdf_text_chunker:
def read_pdf(self, file_url: str) -> str:
logger = logging.getLogger("udf_logger")
logger.info(f"Opening file {file_url}")
with SnowflakeFile.open(file_url, 'rb') as f:
buffer = io.BytesIO(f.readall())
reader = PyPDF2.PdfReader(buffer)
text = ""
for page in reader.pages:
try:
text += page.extract_text().replace('\n', ' ').replace('\0', ' ')
except:
text = "Unable to Extract"
logger.warn(f"Unable to extract from file {file_url}, page {page}")
return text
def process(self, file_url: str):
text = self.read_pdf(file_url)
text_splitter = RecursiveCharacterTextSplitter(
chunk_size = 2000, # Adjust this as needed
chunk_overlap = 300, # Overlap to keep chunks contextual
length_function = len
)
chunks = text_splitter.split_text(text)
df = pd.DataFrame(chunks, columns=['chunk'])
yield from df.itertuples(index=False, name=None)
$$;
次に、 PDF ファイルから解析されたデータを格納するテーブルを作成します。
CREATE OR REPLACE TABLE cortex_search_tutorial_db.public.docs_chunks_table AS
SELECT
relative_path,
build_scoped_file_url(@cortex_search_tutorial_db.public.fomc, relative_path) AS file_url,
-- preserve file title information by concatenating relative_path with the chunk
CONCAT(relative_path, ': ', func.chunk) AS chunk,
'English' AS language
FROM
directory(@cortex_search_tutorial_db.public.fomc),
TABLE(cortex_search_tutorial_db.public.pdf_text_chunker(build_scoped_file_url(@cortex_search_tutorial_db.public.fomc, relative_path))) AS func;
ステップ4: 検索サービスを作成する¶
次の SQL コマンドを実行して、新しいテーブルに対する検索サービスを作成します。
CREATE OR REPLACE CORTEX SEARCH SERVICE cortex_search_tutorial_db.public.fomc_meeting
ON chunk
ATTRIBUTES language
WAREHOUSE = cortex_search_tutorial_wh
TARGET_LAG = '1 hour'
AS (
SELECT
chunk,
relative_path,
file_url,
language
FROM cortex_search_tutorial_db.public.docs_chunks_table
);
このコマンドは、検索結果をフィルタリングする列である attributes
、ウェアハウスとターゲットラグを指定します。検索列は chunk
と指定され、ベーステーブルの複数のテキスト列の連結としてソースクエリで生成されます。ソースクエリの他の列は、検索リクエストに応答して含めることができます。
ステップ5: Streamlitアプリを作成する¶
Python SDK (snowflake
Pythonパッケージを使用)でサービスにクエリすることができます。このチュートリアルでは、 Streamlit in Snowflake アプリケーションでPython SDK を使用する例を示します。
まず、グローバル Snowsight UI ロールが、サービス作成ステップでサービスを作成するために使用したロールと同じであることを確認します。
Snowsight にサインインします。
左側のナビゲーションメニューで Projects » Streamlit を選択します。
+ Streamlit App を選択します。
重要: アプリのロケーションに対応する
cortex_search_tutorial_db
データベースとpublic
スキーマを選択します。Streamlit in Snowflake エディターの左ペインで、 Packages を選択し、
snowflake
(バージョン>= 0.8.0) とsnowflake-ml-python
を追加して、アプリケーションに必要なパッケージをインストールします。サンプルアプリケーションコードを以下のStreamlitアプリに置き換えてください。
import streamlit as st from snowflake.core import Root # requires snowflake>=0.8.0 from snowflake.cortex import Complete from snowflake.snowpark.context import get_active_session """" The available models are subject to change. Check the model availability for the REST API: https://docs.snowflake.com/en/user-guide/snowflake-cortex/cortex-llm-rest-api#model-availability """" MODELS = [ "mistral-large2", "llama3.1-70b", "llama3.1-8b", ] def init_messages(): """ Initialize the session state for chat messages. If the session state indicates that the conversation should be cleared or if the "messages" key is not in the session state, initialize it as an empty list. """ if st.session_state.clear_conversation or "messages" not in st.session_state: st.session_state.messages = [] def init_service_metadata(): """ Initialize the session state for cortex search service metadata. Query the available cortex search services from the Snowflake session and store their names and search columns in the session state. """ if "service_metadata" not in st.session_state: services = session.sql("SHOW CORTEX SEARCH SERVICES;").collect() service_metadata = [] if services: for s in services: svc_name = s["name"] svc_search_col = session.sql( f"DESC CORTEX SEARCH SERVICE {svc_name};" ).collect()[0]["search_column"] service_metadata.append( {"name": svc_name, "search_column": svc_search_col} ) st.session_state.service_metadata = service_metadata def init_config_options(): """ Initialize the configuration options in the Streamlit sidebar. Allow the user to select a cortex search service, clear the conversation, toggle debug mode, and toggle the use of chat history. Also provide advanced options to select a model, the number of context chunks, and the number of chat messages to use in the chat history. """ st.sidebar.selectbox( "Select cortex search service:", [s["name"] for s in st.session_state.service_metadata], key="selected_cortex_search_service", ) st.sidebar.button("Clear conversation", key="clear_conversation") st.sidebar.toggle("Debug", key="debug", value=False) st.sidebar.toggle("Use chat history", key="use_chat_history", value=True) with st.sidebar.expander("Advanced options"): st.selectbox("Select model:", MODELS, key="model_name") st.number_input( "Select number of context chunks", value=5, key="num_retrieved_chunks", min_value=1, max_value=10, ) st.number_input( "Select number of messages to use in chat history", value=5, key="num_chat_messages", min_value=1, max_value=10, ) st.sidebar.expander("Session State").write(st.session_state) def query_cortex_search_service(query, columns = [], filter={}): """ Query the selected cortex search service with the given query and retrieve context documents. Display the retrieved context documents in the sidebar if debug mode is enabled. Return the context documents as a string. Args: query (str): The query to search the cortex search service with. Returns: str: The concatenated string of context documents. """ db, schema = session.get_current_database(), session.get_current_schema() cortex_search_service = ( root.databases[db] .schemas[schema] .cortex_search_services[st.session_state.selected_cortex_search_service] ) context_documents = cortex_search_service.search( query, columns=columns, filter=filter, limit=st.session_state.num_retrieved_chunks ) results = context_documents.results service_metadata = st.session_state.service_metadata search_col = [s["search_column"] for s in service_metadata if s["name"] == st.session_state.selected_cortex_search_service][0].lower() context_str = "" for i, r in enumerate(results): context_str += f"Context document {i+1}: {r[search_col]} \n" + "\n" if st.session_state.debug: st.sidebar.text_area("Context documents", context_str, height=500) return context_str, results def get_chat_history(): """ Retrieve the chat history from the session state limited to the number of messages specified by the user in the sidebar options. Returns: list: The list of chat messages from the session state. """ start_index = max( 0, len(st.session_state.messages) - st.session_state.num_chat_messages ) return st.session_state.messages[start_index : len(st.session_state.messages) - 1] def complete(model, prompt): """ Generate a completion for the given prompt using the specified model. Args: model (str): The name of the model to use for completion. prompt (str): The prompt to generate a completion for. Returns: str: The generated completion. """ return Complete(model, prompt).replace("$", "\$") def make_chat_history_summary(chat_history, question): """ Generate a summary of the chat history combined with the current question to extend the query context. Use the language model to generate this summary. Args: chat_history (str): The chat history to include in the summary. question (str): The current user question to extend with the chat history. Returns: str: The generated summary of the chat history and question. """ prompt = f""" [INST] Based on the chat history below and the question, generate a query that extend the question with the chat history provided. The query should be in natural language. Answer with only the query. Do not add any explanation. <chat_history> {chat_history} </chat_history> <question> {question} </question> [/INST] """ summary = complete(st.session_state.model_name, prompt) if st.session_state.debug: st.sidebar.text_area( "Chat history summary", summary.replace("$", "\$"), height=150 ) return summary def create_prompt(user_question): """ Create a prompt for the language model by combining the user question with context retrieved from the cortex search service and chat history (if enabled). Format the prompt according to the expected input format of the model. Args: user_question (str): The user's question to generate a prompt for. Returns: str: The generated prompt for the language model. """ if st.session_state.use_chat_history: chat_history = get_chat_history() if chat_history != []: question_summary = make_chat_history_summary(chat_history, user_question) prompt_context, results = query_cortex_search_service( question_summary, columns=["chunk", "file_url", "relative_path"], filter={"@and": [{"@eq": {"language": "English"}}]}, ) else: prompt_context, results = query_cortex_search_service( user_question, columns=["chunk", "file_url", "relative_path"], filter={"@and": [{"@eq": {"language": "English"}}]}, ) else: prompt_context, results = query_cortex_search_service( user_question, columns=["chunk", "file_url", "relative_path"], filter={"@and": [{"@eq": {"language": "English"}}]}, ) chat_history = "" prompt = f""" [INST] You are a helpful AI chat assistant with RAG capabilities. When a user asks you a question, you will also be given context provided between <context> and </context> tags. Use that context with the user's chat history provided in the between <chat_history> and </chat_history> tags to provide a summary that addresses the user's question. Ensure the answer is coherent, concise, and directly relevant to the user's question. If the user asks a generic question which cannot be answered with the given context or chat_history, just say "I don't know the answer to that question. Don't saying things like "according to the provided context". <chat_history> {chat_history} </chat_history> <context> {prompt_context} </context> <question> {user_question} </question> [/INST] Answer: """ return prompt, results def main(): st.title(f":speech_balloon: Chatbot with Snowflake Cortex") init_service_metadata() init_config_options() init_messages() icons = {"assistant": "❄️", "user": "👤"} # Display chat messages from history on app rerun for message in st.session_state.messages: with st.chat_message(message["role"], avatar=icons[message["role"]]): st.markdown(message["content"]) disable_chat = ( "service_metadata" not in st.session_state or len(st.session_state.service_metadata) == 0 ) if question := st.chat_input("Ask a question...", disabled=disable_chat): # Add user message to chat history st.session_state.messages.append({"role": "user", "content": question}) # Display user message in chat message container with st.chat_message("user", avatar=icons["user"]): st.markdown(question.replace("$", "\$")) # Display assistant response in chat message container with st.chat_message("assistant", avatar=icons["assistant"]): message_placeholder = st.empty() question = question.replace("'", "") prompt, results = create_prompt(question) with st.spinner("Thinking..."): generated_response = complete( st.session_state.model_name, prompt ) # build references table for citation markdown_table = "###### References \n\n| PDF Title | URL |\n|-------|-----|\n" for ref in results: markdown_table += f"| {ref['relative_path']} | [Link]({ref['file_url']}) |\n" message_placeholder.markdown(generated_response + "\n\n" + markdown_table) st.session_state.messages.append( {"role": "assistant", "content": generated_response} ) if __name__ == "__main__": session = get_active_session() root = Root(session) main()
ステップ6: アプリを試す¶
Streamlit in Snowflake エディターウィンドウの右ペインに、Streamlitアプリのプレビューが表示されます。次のようになります。
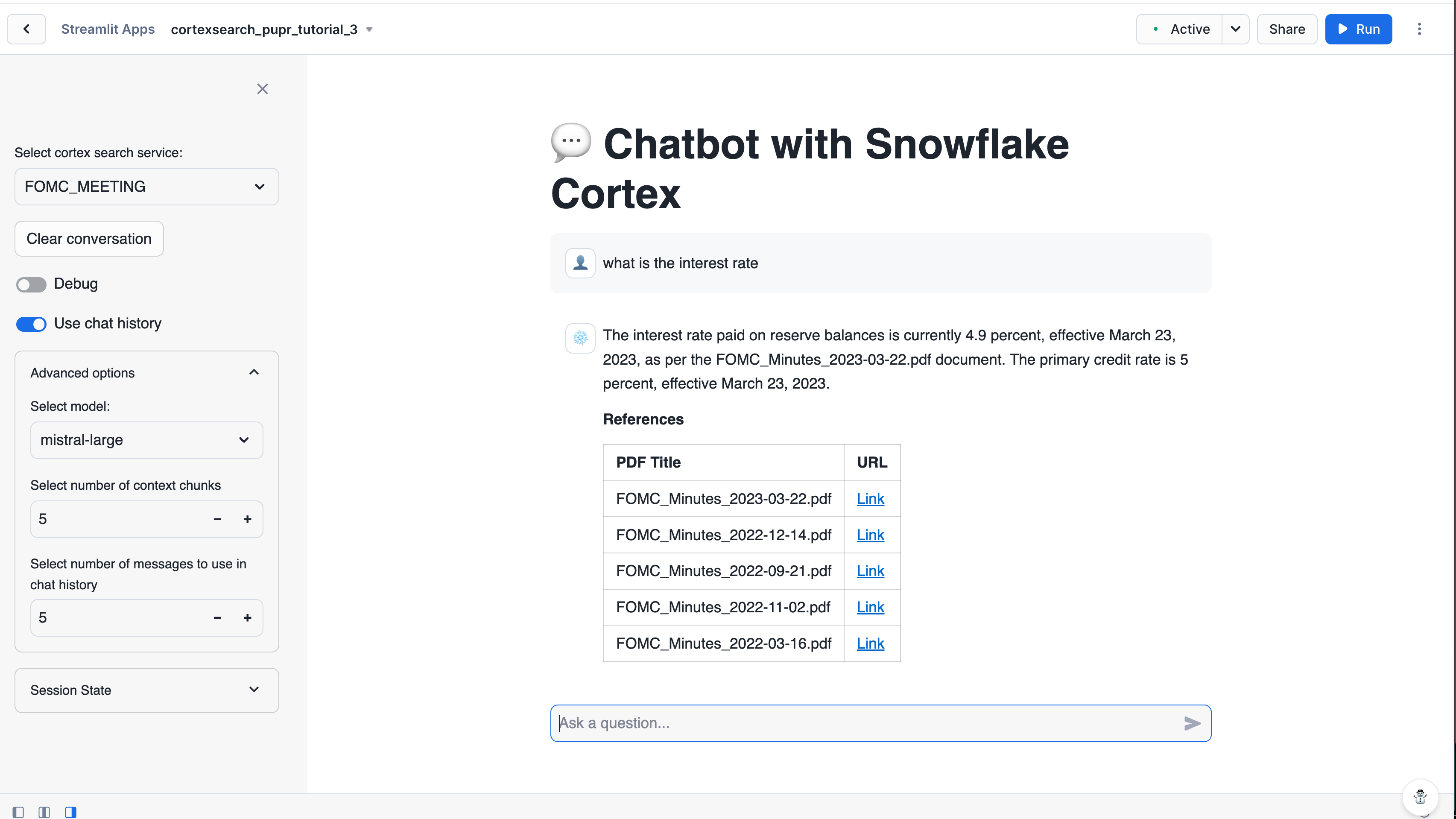
テキストボックスにクエリを入力して、新しいアプリを試してみます。クエリのサンプルは以下の通りです。
- セッション例1: マルチターン質疑応答
How was gpd growth in q4 23?
How was unemployment in the same quarter?
- セッション例2: 複数の文書の要約
How has the fed's view of the market change over the course of 2024?
- セッション例3: 書類に正解が書かれていない場合の棄権
What was janet yellen's opinion about 2024 q1?
ステップ7: クリーンアップする¶
クリーンアップ(オプション)¶
次の DROP <オブジェクト> コマンドを実行して、システムをチュートリアルを開始する前の状態に戻します。
DROP DATABASE IF EXISTS cortex_search_tutorial_db;
DROP WAREHOUSE IF EXISTS cortex_search_tutorial_wh;
データベースをドロップすると、テーブルなどのすべての子データベースオブジェクトが自動的に削除されます。
次のステップ¶
おめでとうございます。Snowflakeで PDF ファイル一式から検索アプリを構築することに成功しました。
追加のリソース¶
以下のリソースを使って学習を続行します。