Container Runtime for ML¶
Overview¶
Container Runtime for ML is a set of preconfigured customizable environments built for machine learning on Snowpark Container Services, covering interactive experimentation and batch ML workloads such as model training, hyperparameter tuning, batch inference and fine tuning. They include the most popular machine learning and deep learning frameworks. Used with Snowflake notebooks, they provide an end-to-end ML experience.
Execution environment¶
The Container Runtime for ML provides an environment populated with packages and libraries that support a wide variety of ML development tasks inside Snowflake. In addition to the pre-installed packages, you can import packages from external sources like public PyPI repositories, or internally-hosted package repositories that provide a list of packages approved for use inside your organization.
Executions of your custom Python ML workloads and supported training APIs occur within Snowpark Container Services, which offers the ability to run on CPU or GPU compute pools. When using the Snowflake ML APIs, the Container Runtime for ML distributes the processing across available resources.
Distributed processing¶
The Snowflake ML modeling and data loading APIs are built on top of Snowflake ML’s distributed processing framework, which maximizes resource utilization by fully leveraging the available compute power. By default, this framework uses all GPUs on multi-GPU nodes, offering significant performance improvements compared to open-source packages and reduces overall runtime.
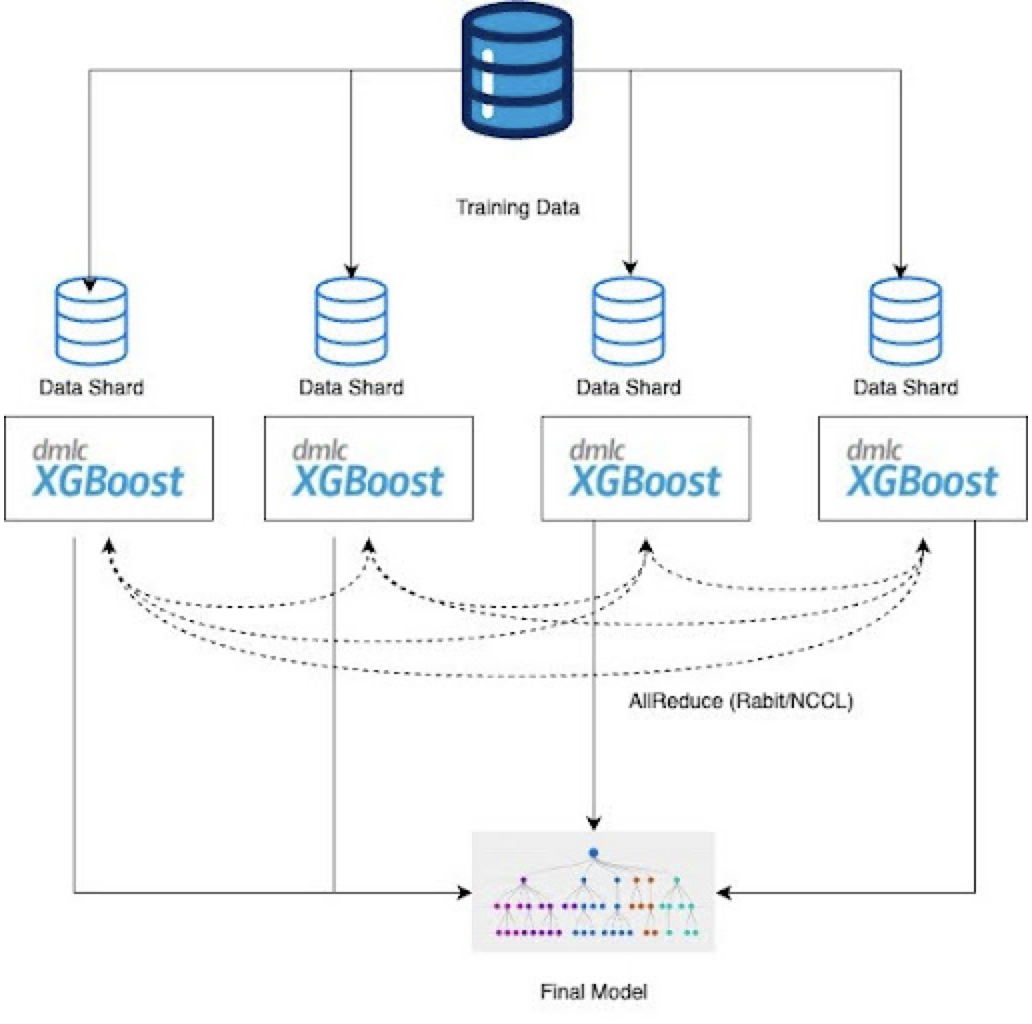
Machine learning workloads, including data loading, are executed in a Snowflake-managed compute environment. The framework allows dynamic scaling of resources based on the specific requirements of the task at hand, such as training models or loading data. The number of resources, including GPU and memory allocation for each task, can be easily configured through the provided APIs.
Optimized data loading¶
The Container Runtime provides a set of data connector APIs that enable connecting Snowflake data sources (including
tables, DataFrames, and Datasets) to popular ML frameworks such as PyTorch and TensorFlow, taking full advantage of
multiple cores or GPUs. Once loaded, the data can be processed using open source packages, or any of the Snowflake ML
APIs, including the distributed versions that are described below. These APIs are found in the snowflake.ml.data
namespace.
The snowflake.ml.data.data_connector.DataConnector
class connects Snowpark DataFrames or Snowflake ML Datasets to
TensorFlow or PyTorch DataSets or Pandas DataFrames. Instantiate a connector using one of the following class methods:
DataConnector.from_dataframe
: Accepts a Snowpark DataFrame.
DataConnector.from_dataset
: Accepts a Snowflake ML Dataset, specified by name and version.
DataConnector.from_sources
: Accepts list of sources, each of which can be a DataFrame or a Dataset.
Once you have instantiated the connector (calling the instance, for example, data_connector
), call the following
methods to produce the desired kind of output.
data_connector.to_tf_dataset
: Returns a TensorFlow Dataset suitable for use with TensorFlow.data_connector.to_torch_dataset
: Returns a PyTorch Dataset suitable for use with PyTorch.
For more information on these APIs, see the Snowflake ML API reference.
Building with open source¶
With the foundational CPU and GPU images that come pre-populated with popular ML packages, and the flexibility to
install additional libraries using pip
, users can employ familiar and innovative open source frameworks inside Snowflake
Notebooks, without moving data out of Snowflake. You can scale processing by using Snowflake’s distributed
APIs for data loading, training, and hyperparameter optimization, with the familiar APIs of popular OSS
packages, with small changes to the interface to allow for scaling configurations.
The following code illustrates creating an XGBoost classifier using these APIs:
from snowflake.snowpark.context import get_active_session
from snowflake.ml.data.data_connector import DataConnector
import pandas as pd
import xgboost as xgb
from sklearn.model_selection import train_test_split
session = get_active_session()
# Use the DataConnector API to pull in large data efficiently
df = session.table("my_dataset")
pandas_df = DataConnector.from_dataframe(df).to_pandas()
# Build with open source
X = df_pd[['feature1', 'feature2']]
y = df_pd['label']
# Split data into test and train in memory
X_train, X_test, y_train, y_test = train_test_split(X, y, test_size=0.15, random_state=34)
# Train in memory
model = xgb.XGBClassifier()
model.fit(X_train, y_train)
# Predict
y_pred = model.predict(X_test)
Optimized training¶
Container Runtime for ML offers a set of distributed training APIs, including distributed versions of LightGBM, PyTorch,
and XGBoost, that take full advantage of the available resources in the container environment. These are found in the
snowflake.ml.modeling.distributors
namespace. The APIs of the distributed classes are similar to those of the
standard versions.
For more information on these APIs, see the API reference.
XGBoost¶
The primary XGBoost class is snowflake.ml.modeling.distributors.xgboost.XGBEstimator
. Related classes include:
snowflake.ml.modeling.distributors.xgboost.XGBScalingConfig
For an example of working with this API, see the XGBoost on GPU example notebook in the Snowflake Container Runtime for ML GitHub repository.
LightGBM¶
The primary LightGBM class is snowflake.ml.modeling.distributors.lightgbm.LightGBMEstimator
. Related classes include:
snowflake.ml.modeling.distributors.lightgbm.LightGBMScalingConfig
For an example of working with this API, see the LightGBM on GPU example notebook in the Snowflake Container Runtime for ML GitHub repository.
PyTorch¶
The primary PyTorch class is snowflake.ml.modeling.distributors.pytorch.PyTorchDistributor
. Related classes and functions include:
snowflake.ml.modeling.distributors.pytorch.WorkerResourceConfig
snowflake.ml.modeling.distributors.pytorch.PyTorchScalingConfig
snowflake.ml.modeling.distributors.pytorch.Context
snowflake.ml.modeling.distributors.pytorch.get_context
For an example of working with this API, see the PyTorch on GPU example notebook in the Snowflake Container Runtime for ML GitHub repository.
Snowflake ML modeling APIs¶
When Snowflake ML’s modeling APIs are used in a Notebook, all execution happens on the container runtime instead of on
the query warehouse, with the exception of the snowflake.ml.modeling.preprocessing
APIs, which are executed in the query warehouse.
Limitations¶
The Snowflake ML Modeling API supports only
predict
,predict_proba
, andpredict_log_proba
inference methods on Container Runtime for ML. Other methods run in the query warehouse.The Snowflake ML Modeling API supports only sklearn-compatible pipelines on Container Runtime for ML.
Snowflake ML Modeling API does not support preprocessing or metrics classes on Container Runtime for ML. These APIs run in the query warehouse.
The
fit
,predict
, andscore
methods are executed on Container Runtime for ML. Other Snowflake ML methods run in the query warehouse.sample_weight_cols
is not supported for XGBoost or LightGBM models.
Container Runtime image specification¶
You can choose between CPU or GPU image types when creating a notebook to run on Container Runtime. Both images come pre-installed with popular ML frameworks like scikit-learn and PyTorch. You can also use Snowpark ML and everything it includes.
Full list for CPU v1 image¶
This table is a full list of Python packages pre-installed on the CPU v1 image:
Package |
Version |
---|---|
absl-py |
1.4.0 |
aiobotocore |
2.7.0 |
aiohttp |
3.9.5 |
aiohttp-cors |
0.7.0 |
aioitertools |
0.12.0 |
aiosignal |
1.2.0 |
aiosignal |
1.3.1 |
altair |
5.4.1 |
annotated-types |
0.6.0 |
anyio |
3.5.0 |
appdirs |
1.4.4 |
arviz |
0.17.1 |
asn1crypto |
1.5.1 |
asttokens |
2.0.5 |
async-timeout |
4.0.3 |
async-timeout |
4.0.3 |
atpublic |
4.0 |
attrs |
23.1.0 |
attrs |
23.2.0 |
backoff |
2.2.1 |
bayesian-optimization |
1.5.1 |
blinker |
1.6.2 |
botocore |
1.31.64 |
bottleneck |
1.3.7 |
brotli |
1.0.9 |
cachetools |
5.3.3 |
causalpy |
0.4.0 |
certifi |
2024.8.30 |
cffi |
1.16.0 |
charset-normalizer |
3.3.2 |
click |
8.1.7 |
clikit |
0.6.2 |
cloudpickle |
2.2.1 |
cmdstanpy |
1.2.4 |
colorama |
0.4.6 |
colorful |
0.5.4 |
cons |
0.4.6 |
contourpy |
1.2.0 |
crashtest |
0.3.1 |
cryptography |
42.0.8 |
cycler |
0.11.0 |
datasets |
2.16.1 |
decorator |
5.1.1 |
deprecated |
1.2.13 |
dill |
0.3.7 |
distlib |
0.3.8 |
etuples |
0.3.9 |
evaluate |
0.4.2 |
exceptiongroup |
1.2.0 |
executing |
0.8.3 |
filelock |
3.13.1 |
flask |
3.0.3 |
fonttools |
4.51.0 |
frozenlist |
1.4.0 |
frozenlist |
1.4.1 |
fsspec |
2023.10.0 |
gitdb |
4.0.7 |
gitpython |
3.1.41 |
gmpy2 |
2.1.2 |
google-api-core |
2.19.1 |
google-auth |
2.29.0 |
googleapis-common-protos |
1.63.2 |
graphviz |
0.20.1 |
grpcio |
1.66.1 |
grpcio-tools |
1.62.3 |
gunicorn |
22.0.0 |
h5netcdf |
1.2.0 |
h5py |
3.11.0 |
holidays |
0.57 |
httpstan |
4.13.0 |
huggingface-hub |
0.24.6 |
idna |
3.6 |
idna |
3.7 |
importlib-metadata |
6.11.0 |
importlib-resources |
6.4.5 |
ipython |
8.27.0 |
itsdangerous |
2.2.0 |
jedi |
0.19.1 |
jinja2 |
3.1.4 |
jmespath |
1.0.1 |
joblib |
1.4.2 |
jsonschema |
4.19.2 |
jsonschema-specifications |
2023.7.1 |
kiwisolver |
1.4.4 |
lightgbm |
3.3.5 |
lightgbm-ray |
0.1.9 |
logical-unification |
0.4.6 |
markdown-it-py |
2.2.0 |
markupsafe |
2.1.3 |
marshmallow |
3.22.0 |
matplotlib |
3.8.4 |
matplotlib-inline |
0.1.6 |
mdurl |
0.1.0 |
minikanren |
1.0.3 |
mkl-fft |
1.3.10 |
mkl-random |
1.2.7 |
mkl-service |
2.4.0 |
mlruntimes-client |
0.2.0 |
mlruntimes-service |
0.2.0 |
modin |
0.31.0 |
mpmath |
1.3.0 |
msgpack |
1.0.3 |
multidict |
6.0.4 |
multidict |
6.0.5 |
multipledispatch |
0.6.0 |
multiprocess |
0.70.15 |
narwhals |
1.8.4 |
networkx |
3.3 |
nltk |
3.9.1 |
numexpr |
2.8.7 |
numpy |
1.24.3 |
opencensus |
0.11.3 |
opencensus-context |
0.1.3 |
opencv-python |
4.10.0.84 |
opentelemetry-api |
1.23.0 |
opentelemetry-exporter-otlp-proto-common |
1.23.0 |
opentelemetry-exporter-otlp-proto-grpc |
1.25.0 |
opentelemetry-proto |
1.23.0 |
opentelemetry-sdk |
1.23.0 |
opentelemetry-semantic-conventions |
0.44b0 |
packaging |
23.1 |
pandas |
2.2.3 |
parso |
0.8.3 |
pastel |
0.2.1 |
patsy |
0.5.6 |
pexpect |
4.8.0 |
pillow |
9.5.0 |
pip |
24.2 |
platformdirs |
2.6.2 |
plotly |
5.22.0 |
ply |
3.11 |
prometheus-client |
0.20.0 |
prompt-toolkit |
3.0.43 |
prophet |
1.1.5 |
proto-plus |
1.24.0 |
protobuf |
4.24.4 |
psutil |
5.9.0 |
ptyprocess |
0.7.0 |
pure-eval |
0.2.2 |
pyarrow |
15.0.0 |
pyarrow-hotfix |
0.6 |
pyasn1 |
0.4.8 |
pyasn1-modules |
0.2.8 |
pycparser |
2.21 |
pydantic |
2.8.2 |
pydantic-core |
2.20.1 |
pydeck |
0.9.1 |
pygments |
2.15.1 |
pyjwt |
2.8.0 |
pylev |
1.4.0 |
pymc |
5.16.1 |
pympler |
1.1 |
pyopenssl |
24.2.1 |
pyparsing |
3.0.9 |
pyqt5 |
5.15.10 |
pyqt5-sip |
12.13.0 |
pysimdjson |
6.0.2 |
pysocks |
1.7.1 |
pystan |
3.10.0 |
pytensor |
2.13.1 |
pytensor |
2.23.0 |
python-dateutil |
2.8.3+snowflake1 |
pytimeparse |
1.1.8 |
pytz |
2024.1 |
pytz-deprecation-shim |
0.1.0.post0 |
pyyaml |
6.0.1 |
ray |
2.10.0 |
referencing |
0.30.2 |
regex |
2024.7.24 |
requests |
2.32.3 |
retrying |
1.3.4 |
rich |
13.7.1 |
rpds-py |
0.10.6 |
rsa |
4.7.2 |
s3fs |
2023.10.0 |
safetensors |
0.4.4 |
scikit-learn |
1.3.0 |
scipy |
1.13.1 |
seaborn |
0.13.2 |
setproctitle |
1.2.2 |
setuptools |
70.0.0 |
sip |
6.7.12 |
six |
1.16.0 |
smart-open |
5.2.1 |
smmap |
4.0.0 |
sniffio |
1.3.0 |
snowbooks |
1.46.0 |
snowflake |
0.12.1 |
snowflake-connector-python |
3.12.0 |
snowflake-core |
0.12.1 |
snowflake-legacy |
0.12.1 |
snowflake-ml-python |
1.6.2 |
snowflake-snowpark-python |
1.18.0 |
snowflake-telemetry-python |
0.5.0 |
sortedcontainers |
2.4.0 |
sqlparse |
0.5.1 |
stack-data |
0.2.0 |
stanio |
0.5.1 |
statsmodels |
0.14.2 |
streamlit |
1.26.0 |
sympy |
1.13.2 |
tenacity |
8.2.3 |
tensorboardx |
2.6.2.2 |
threadpoolctl |
3.5.0 |
tokenizers |
0.15.1 |
toml |
0.10.2 |
tomli |
2.0.1 |
tomlkit |
0.11.1 |
toolz |
0.12.0 |
torch |
2.3.0 |
tornado |
6.4.1 |
tqdm |
4.66.4 |
traitlets |
5.14.3 |
transformers |
4.36.0 |
typing-extensions |
4.12.2 |
tzdata |
2024.2 |
tzlocal |
4.3.1 |
unicodedata2 |
15.1.0 |
urllib3 |
2.0.7 |
validators |
0.34.0 |
virtualenv |
20.17.1 |
watchdog |
5.0.3 |
wcwidth |
0.2.5 |
webargs |
8.6.0 |
werkzeug |
3.0.3 |
wheel |
0.43.0 |
wrapt |
1.14.1 |
xarray |
2023.6.0 |
xarray-einstats |
0.6.0 |
xgboost |
1.7.6 |
xgboost-ray |
0.1.19 |
xxhash |
2.0.2 |
yarl |
1.11.0 |
yarl |
1.9.4 |
zipp |
3.17.0 |
Full list for GPU v1 image¶
This table is a full list of Python packages pre-installed on the GPU v1 image:
Package |
Version |
---|---|
absl-py |
1.4.0 |
accelerate |
0.34.2 |
aiobotocore |
2.7.0 |
aiohttp |
3.9.5 |
aiohttp-cors |
0.7.0 |
aioitertools |
0.12.0 |
aiosignal |
1.2.0 |
aiosignal |
1.3.1 |
altair |
5.4.1 |
annotated-types |
0.6.0 |
anyio |
3.5.0 |
appdirs |
1.4.4 |
arviz |
0.17.1 |
asn1crypto |
1.5.1 |
asttokens |
2.0.5 |
async-timeout |
4.0.3 |
async-timeout |
4.0.3 |
atpublic |
4.0 |
attrs |
23.1.0 |
attrs |
23.2.0 |
backoff |
2.2.1 |
bayesian-optimization |
1.5.1 |
blinker |
1.6.2 |
botocore |
1.31.64 |
bottleneck |
1.3.7 |
brotli |
1.0.9 |
cachetools |
5.3.3 |
causalpy |
0.4.0 |
certifi |
2024.8.30 |
cffi |
1.16.0 |
charset-normalizer |
3.3.2 |
click |
8.1.7 |
clikit |
0.6.2 |
cloudpickle |
2.0.0 |
cmake |
3.30.3 |
cmdstanpy |
1.2.4 |
colorama |
0.4.6 |
colorful |
0.5.4 |
cons |
0.4.6 |
contourpy |
1.2.0 |
crashtest |
0.3.1 |
cryptography |
42.0.8 |
cycler |
0.11.0 |
datasets |
2.16.1 |
decorator |
5.1.1 |
deprecated |
1.2.13 |
dill |
0.3.7 |
diskcache |
5.6.3 |
distlib |
0.3.8 |
distro |
1.9.0 |
etuples |
0.3.9 |
evaluate |
0.4.2 |
exceptiongroup |
1.2.0 |
executing |
0.8.3 |
fastapi |
0.115.0 |
filelock |
3.13.1 |
flask |
3.0.3 |
fonttools |
4.51.0 |
frozenlist |
1.4.0 |
frozenlist |
1.4.1 |
fsspec |
2023.10.0 |
gitdb |
4.0.7 |
gitpython |
3.1.41 |
gmpy2 |
2.1.2 |
google-api-core |
2.19.1 |
google-auth |
2.29.0 |
googleapis-common-protos |
1.63.2 |
graphviz |
0.20.1 |
grpcio |
1.66.1 |
grpcio-tools |
1.62.3 |
gunicorn |
22.0.0 |
h11 |
0.14.0 |
h5netcdf |
1.2.0 |
h5py |
3.11.0 |
holidays |
0.57 |
httpcore |
1.0.5 |
httpstan |
4.13.0 |
httptools |
0.6.1 |
httpx |
0.27.2 |
huggingface-hub |
0.24.6 |
idna |
3.6 |
idna |
3.7 |
importlib-metadata |
6.11.0 |
importlib-resources |
6.4.5 |
interegular |
0.3.3 |
ipython |
8.27.0 |
itsdangerous |
2.2.0 |
jedi |
0.19.1 |
jinja2 |
3.1.4 |
jiter |
0.5.0 |
jmespath |
1.0.1 |
joblib |
1.4.2 |
jsonschema |
4.19.2 |
jsonschema-specifications |
2023.7.1 |
kiwisolver |
1.4.4 |
lark |
1.2.2 |
lightgbm |
4.5.0 |
lightgbm-ray |
0.1.9 |
llvmlite |
0.43.0 |
lm-format-enforcer |
0.10.3 |
logical-unification |
0.4.6 |
markdown-it-py |
2.2.0 |
markupsafe |
2.1.3 |
marshmallow |
3.22.0 |
matplotlib |
3.8.4 |
matplotlib-inline |
0.1.6 |
mdurl |
0.1.0 |
minikanren |
1.0.3 |
mkl-fft |
1.3.10 |
mkl-random |
1.2.7 |
mkl-service |
2.4.0 |
mlruntimes-client |
0.2.0 |
mlruntimes-service |
0.2.0 |
modin |
0.31.0 |
mpmath |
1.3.0 |
msgpack |
1.0.3 |
multidict |
6.0.4 |
multidict |
6.0.5 |
multipledispatch |
0.6.0 |
multiprocess |
0.70.15 |
narwhals |
1.8.4 |
nest-asyncio |
1.6.0 |
networkx |
3.3 |
ninja |
1.11.1.1 |
nltk |
3.9.1 |
numba |
0.60.0 |
numexpr |
2.8.7 |
numpy |
1.24.3 |
nvidia-cublas-cu12 |
12.1.3.1 |
nvidia-cuda-cupti-cu12 |
12.1.105 |
nvidia-cuda-nvrtc-cu12 |
12.1.105 |
nvidia-cuda-runtime-cu12 |
12.1.105 |
nvidia-cudnn-cu12 |
8.9.2.26 |
nvidia-cufft-cu12 |
11.0.2.54 |
nvidia-curand-cu12 |
10.3.2.106 |
nvidia-cusolver-cu12 |
11.4.5.107 |
nvidia-cusparse-cu12 |
12.1.0.106 |
nvidia-ml-py |
12.560.30 |
nvidia-nccl-cu12 |
2.20.5 |
nvidia-nvjitlink-cu12 |
12.6.68 |
nvidia-nvtx-cu12 |
12.1.105 |
openai |
1.50.1 |
opencensus |
0.11.3 |
opencensus-context |
0.1.3 |
opencv-python |
4.10.0.84 |
opentelemetry-api |
1.23.0 |
opentelemetry-exporter-otlp-proto-common |
1.23.0 |
opentelemetry-exporter-otlp-proto-grpc |
1.25.0 |
opentelemetry-proto |
1.23.0 |
opentelemetry-sdk |
1.23.0 |
opentelemetry-semantic-conventions |
0.44b0 |
outlines |
0.0.46 |
packaging |
23.1 |
pandas |
2.2.3 |
parso |
0.8.3 |
pastel |
0.2.1 |
patsy |
0.5.6 |
peft |
0.5.0 |
pexpect |
4.8.0 |
pillow |
9.5.0 |
pip |
24.2 |
platformdirs |
2.6.2 |
plotly |
5.22.0 |
ply |
3.11 |
prometheus-client |
0.20.0 |
prometheus-fastapi-instrumentator |
7.0.0 |
prompt-toolkit |
3.0.43 |
prophet |
1.1.5 |
proto-plus |
1.24.0 |
protobuf |
4.24.4 |
psutil |
5.9.0 |
ptyprocess |
0.7.0 |
pure-eval |
0.2.2 |
py-cpuinfo |
9.0.0 |
pyairports |
2.1.1 |
pyarrow |
15.0.0 |
pyarrow-hotfix |
0.6 |
pyasn1 |
0.4.8 |
pyasn1-modules |
0.2.8 |
pycountry |
24.6.1 |
pycparser |
2.21 |
pydantic |
2.8.2 |
pydantic-core |
2.20.1 |
pydeck |
0.9.1 |
pygments |
2.15.1 |
pyjwt |
2.8.0 |
pylev |
1.4.0 |
pymc |
5.16.1 |
pympler |
1.1 |
pyopenssl |
24.2.1 |
pyparsing |
3.0.9 |
pyqt5 |
5.15.10 |
pyqt5-sip |
12.13.0 |
pysimdjson |
6.0.2 |
pysocks |
1.7.1 |
pystan |
3.10.0 |
pytensor |
2.13.1 |
pytensor |
2.23.0 |
python-dateutil |
2.8.3+snowflake1 |
python-dotenv |
1.0.1 |
pytimeparse |
1.1.8 |
pytz |
2024.1 |
pytz-deprecation-shim |
0.1.0.post0 |
pyyaml |
6.0.1 |
pyzmq |
26.2.0 |
ray |
2.10.0 |
referencing |
0.30.2 |
regex |
2024.7.24 |
requests |
2.32.3 |
retrying |
1.3.4 |
rich |
13.7.1 |
rpds-py |
0.10.6 |
rsa |
4.7.2 |
s3fs |
2023.10.0 |
safetensors |
0.4.4 |
scikit-learn |
1.3.0 |
scipy |
1.9.3 |
seaborn |
0.13.2 |
sentencepiece |
0.1.99 |
setproctitle |
1.2.2 |
setuptools |
70.0.0 |
sip |
6.7.12 |
six |
1.16.0 |
smart-open |
5.2.1 |
smmap |
4.0.0 |
sniffio |
1.3.0 |
snowbooks |
1.46.0 |
snowflake |
0.12.1 |
snowflake-connector-python |
3.12.0 |
snowflake-core |
0.12.1 |
snowflake-legacy |
0.12.1 |
snowflake-ml-python |
1.6.2 |
snowflake-snowpark-python |
1.18.0 |
snowflake-telemetry-python |
0.5.0 |
sortedcontainers |
2.4.0 |
sqlparse |
0.5.1 |
stack-data |
0.2.0 |
stanio |
0.5.1 |
starlette |
0.38.6 |
statsmodels |
0.14.2 |
streamlit |
1.26.0 |
sympy |
1.13.2 |
tenacity |
8.2.3 |
tensorboardx |
2.6.2.2 |
threadpoolctl |
3.5.0 |
tiktoken |
0.7.0 |
tokenizers |
0.20.0 |
toml |
0.10.2 |
tomli |
2.0.1 |
tomlkit |
0.11.1 |
toolz |
0.12.0 |
torch |
2.3.1 |
torchvision |
0.18.1 |
tornado |
6.4.1 |
tqdm |
4.66.4 |
traitlets |
5.14.3 |
transformers |
4.45.1 |
triton |
2.3.1 |
typing-extensions |
4.12.2 |
tzdata |
2024.2 |
tzlocal |
4.3.1 |
unicodedata2 |
15.1.0 |
urllib3 |
2.0.7 |
uvicorn |
0.31.0 |
uvloop |
0.20.0 |
validators |
0.34.0 |
virtualenv |
20.17.1 |
vllm |
0.5.3.post1 |
vllm-flash-attn |
2.5.9.post1 |
watchdog |
5.0.3 |
watchfiles |
0.24.0 |
wcwidth |
0.2.5 |
webargs |
8.6.0 |
websockets |
13.1 |
werkzeug |
3.0.3 |
wheel |
0.43.0 |
wrapt |
1.14.1 |
xarray |
2023.6.0 |
xarray-einstats |
0.6.0 |
xformers |
0.0.27 |
xgboost |
1.7.6 |
xgboost-ray |
0.1.19 |
xxhash |
2.0.2 |
yarl |
1.11.0 |
yarl |
1.9.4 |
zipp |
3.17.0 |
Next steps¶
To try the notebook using Container Runtime for ML, see Notebooks on Container Runtime for ML.