Snowflake Feature Store¶
Note
The Snowflake Feature Store API is available in the Snowpark ML Python package (snowflake-ml-python
) v1.5.0 and later.
The Snowflake Feature Store lets data scientists and ML engineers create, maintain, and use ML features in data science and ML workloads, all within Snowflake.
Generically, features are data elements used as inputs to a machine learning model. Many columns in a dataset, such as temperature or attendance, can be used as features as-is. In other cases, a column can be made more useful for training via preprocessing and transformation. For example, you might derive a day-of-week feature from a timestamp to allow the model to detect weekly patterns. Other common feature transformations involve aggregating, differentiating, or time-shifting data. Feature engineering is the process of deciding what features are needed by your models and defining how they will be derived from the raw data.
A feature store lets you standardize commonly used feature transformations in a central repository, enabling reuse, helping to reduce duplication of data and effort, and improving productivity. It also helps maintain features by updating them on new source data, always providing correct, consistent, and fresh features in a single source of truth. By cultivating consistency in how features are extracted from raw data, a feature store can also help to make your production ML pipelines more robust.
The Snowflake Feature Store is designed to make creating, storing, and managing features for data science and machine learning workloads easier and more efficient. Hosted natively inside Snowflake, the Snowflake Feature Store provides the following advantages:
Your data remains secure, completely under your control and governance, and never leaves Snowflake.
The Snowsight Feature Store UI makes it easy to search for and discover features.
Access is managed with fine-grained role-based access control.
Key benefits of the Snowflake Feature Store include support for:
Both batch and streaming data, with efficient automatic updates as new data arrives
Backfill and point-in-time correct features with ASOF JOIN
Feature transformations authored in Python or SQL
Automatic update and refresh of feature values from source data with Snowflake managed Feature Views
Ability to use user-managed feature pipelines with external tools such as dbt
The Snowflake Feature Store is fully integrated with the Snowflake Model Registry and other Snowflake ML features for end-to-end production ML.
The following illustration shows how the Snowflake Feature Store fits into a machine learning pipeline:
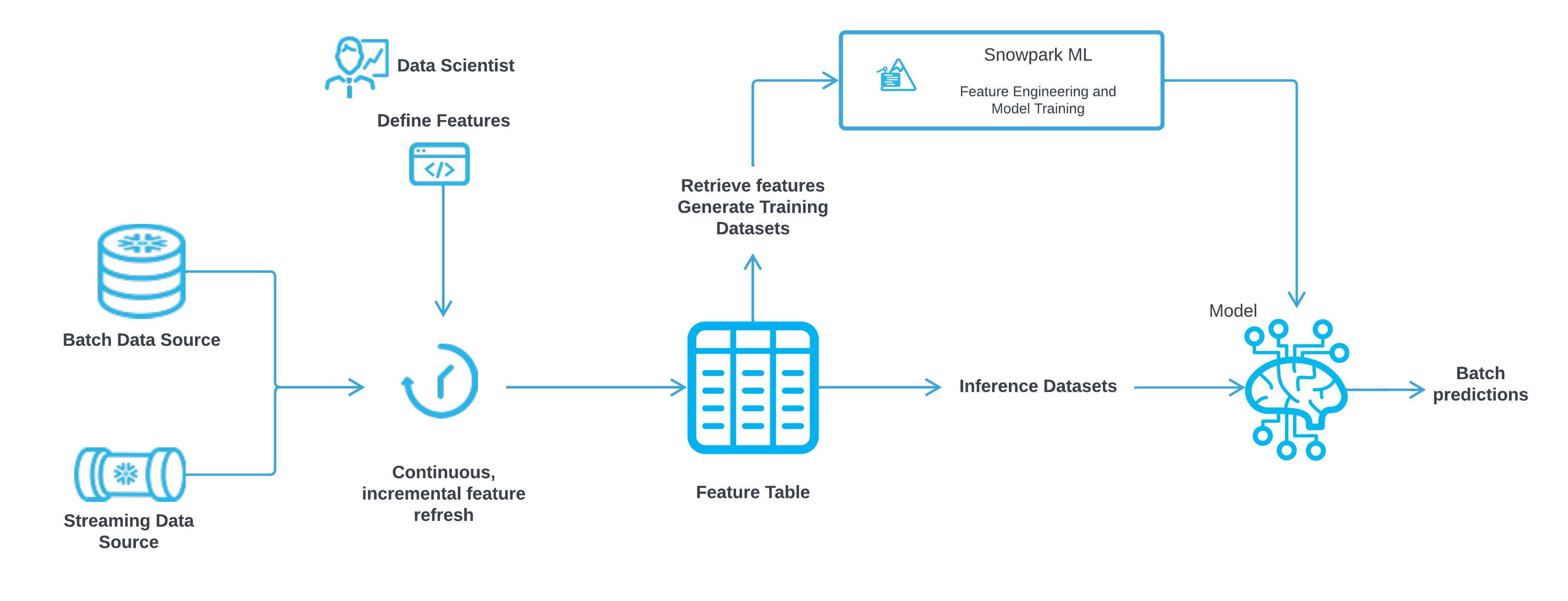
Raw data can be obtained in batch from tables or views or from streaming data sources.
The raw data is then transformed by features defined by data engineers, resulting in a feature table.
The feature table can be used to generate training datasets used for training models in Snowpark ML, or to enrich test data used by the model to make predictions.
How does it work?¶
Note
A feature store in Snowflake is simply a schema. You can create a new schema to use as a feature store, or use an existing one.
A feature store contains feature views. A feature view encapsulates a Python or SQL pipeline for transforming raw data into one or more related features. All features defined in a feature view are refreshed from the source data at the same time.
Tip
Users who have access to more than one feature store can combine feature views from multiple feature stores to create training and inference datasets.
The Snowflake Feature Store supports two kinds of feature views:
Snowflake-managed: The Snowflake Feature Store refreshes the features in the feature view for you, incrementally and efficiently, on a schedule you specify.
External: Some other process outside of the feature store maintains the features in the feature view. This type of feature view is intended for use with tools such as dbt.
Feature views are organized in the feature store according to the entities to which they apply. An entity is a higher-level abstraction that represents the subject matter of a feature. For example, in a feature store for a movie streaming service, the main entities might be users and movies. Raw movie data and user activity data can be converted into useful features such as per-movie viewing time and user session length, and the feature views containing these features can be tagged with relevant entities.
Back-end data model¶
Feature store objects are implemented as Snowflake objects. All feature store objects are therefore subject to Snowflake access control rules.
Feature store object |
Snowflake object |
---|---|
feature store |
|
feature view |
|
entity |
|
feature |
column in a dynamic table or in a view |
Properties of feature views (such as name and entity) are implemented as tags on dynamic tables or views.
You can query or manipulate the Snowflake objects using SQL. Changes you make via SQL are reflected in the Python API and vice versa.
Tip
All objects of a Snowflake Feature Store are stored in the feature store’s schema. To completely delete a feature store, make sure the schema doesn’t contain any other resources, and then drop the schema.
Getting started¶
Note
The Snowflake Feature Store Python API is part of the Snowpark ML Python package, snowflake-ml-python
. You can use
it on your local system in your preferred Python IDE or in a Snowsight worksheet or notebook. For details, see
Python APIs for Snowflake ML.
Begin your journey with Introduction to the Snowflake Feature Store for an introduction to Snowflake Feature Store concepts. Then follow up with additional Snowflake quickstarts, including:
Develop and Manage ML Models with the Snowflake Feature Store and Model Registry. This is an end-to-end ML development cycle demo with the Feature Store and the Model Registry.
Getting Started with the Snowflake Feature Store API. This is an overview of Feature Store Python APIs.
Advanced Guide to the Snowflake Feature Store. This is a more advanced example of Feature Store and pipelines.
Getting Started with Snowflake Feature Store and dbt. This demonstrates how to register features from DBT pipeline into Snowflake Feature Store.
See Common feature and query patterns for examples of specific types of feature transformations.
Note
These are only shown as examples, and following along with the example may require additional rights to third-party data, products, or services that are not owned or provided by Snowflake. Snowflake does not guarantee the accuracy of these examples.